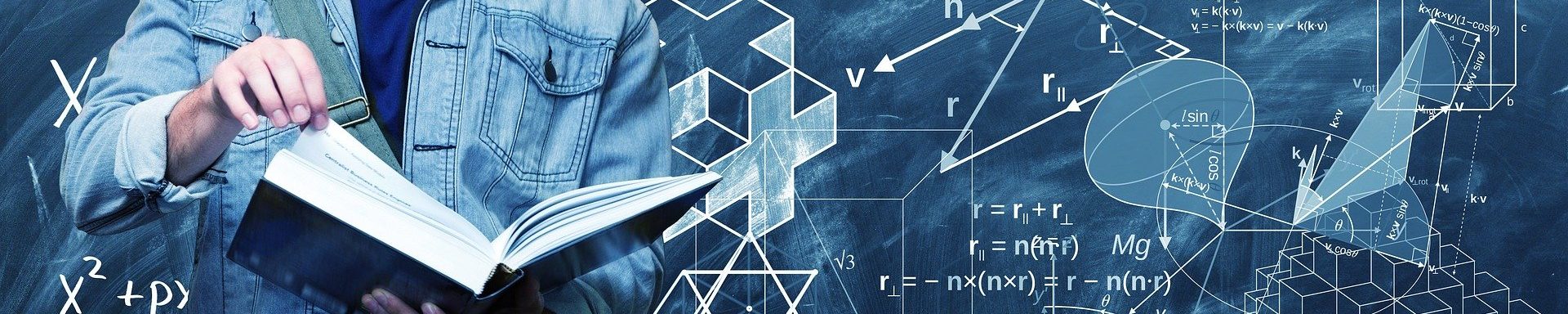
Data Literacy
Notes:
- Students who have declared a primary major in Data Science and Economics before the stipulated deadline for each Academic Year (AY) will be pre-allocated DSE1101 which can fulfil the Data Literacy requirement. DSE1101 can only be read by students in the Data Science and Economics programme.
- Students who have declared a primary major in Data Science and Analytics or Statistics will be pre-allocated their respective gateway courses which can fulfil the Data Literacy requirement.
- The following students will be pre-allocated GEA1000:
- Students who did not declare a primary major in Data Science and Economics before the stipulated deadline for each AY
- Students who did not declare a primary major in Data Science and Analytics or Statistics
- Students who did not declare a second major/minor in Data Analytics/Statistics.
However, if students would like to read ST1131/DSA1101/BT1101 to fulfil the Data Literacy requirements, they can drop GEA1000 and select ST1131/DSA1101/BT1101 instead. Please appeal via the ModReg system under "Drop Lec/Tut allocated by admin". More details can be found here.
- Students reading the primary Major/Second Major/Minor in Statistics are advised to read ST1131 instead of GEA1000 to fulfil the Data Literacy requirement.
- Students reading the primary Major in Data Science and Analytics or Second Major in Data Analytics are advised to read DSA1101 instead of GEA1000 to fulfil the Data Literacy requirement.
- Students reading the Data Science and Economics Cross-Disciplinary Programme are advised to read DSE1101 instead of GEA1000 to fulfil the Data Literacy requirement.
- IE1111R Industrial and Systems Engineering Principles and Practice I can only be read by students in the Industrial and Systems Engineering programme under the College of Design and Engineering to fulfil the Data Literacy pillar.